Information obtained from structural health monitoring (SHM) systems aims to issue alerts for taking further action against potential hazards posed to structural integrity. Furthermore, in wireless SHM systems, embedded computing is often leveraged towards conducting SHM tasks on board wireless sensor nodes so as to avoid the power consuming transmission of entire data sets. The consequent scarcity of raw SHM data renders manual detection of anomalies, such as sensor faults, within the data burdensome. A sensor fault is usually caused by malfunctions or by external factors, resulting in an error and, eventually, in SHM system failure.
To ensure reliable operation of wireless SHM systems, sensor faults need to be effectively handled. Referred to as “fault diagnosis”, managing sensor faults encompasses four stages:
- Fault detection (anomalies in SHM data are recognized)
- Fault isolation (exact location of faults is determined)
- Fault identification (the type of fault is determined)
- Fault accommodation (involves corrective action to alleviate the fault effects)
Fault diagnosis approaches are usually based on comparisons between virtual sensor outputs and actual sensor outputs. In this research, virtual sensor outputs are obtained by exploiting the abundance of structural response data and the inherent correlations between responses from different structural locations (analytical redundancy). The correlation between structural response data sets is approximated by surrogate models.
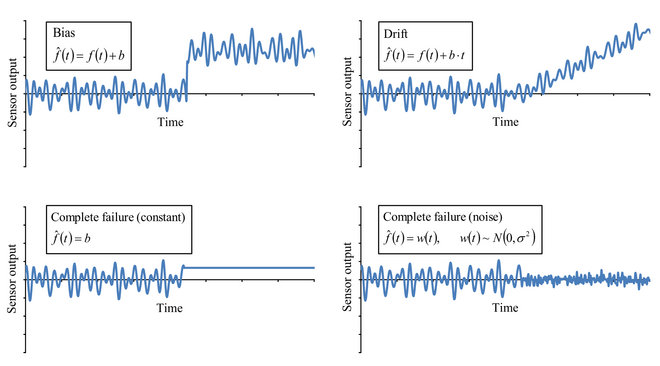
Figure 1: Various sensor fault types
Project-related publications
- Steiner, M., Fritz, H., Thiebes, L. & Dragos, K., 2018. Fault diagnosis in wireless structural health monitoring systems based on support vector regression. In: 30. Forum Bauinformatik. Weimar, 19.09.2018.
- Steiner, M., Legatiuk, D. & Smarsly, K., 2019. A support vector regression-based approach towards decentralized fault diagnosis in wireless structural health monitoring systems. In: The 12th International Workshop on Structural Health Monitoring (IWSHM). Stanford, CA, USA, 10.09.2019.
- Fritz, H., Peralta, J., Legatiuk, D., Steiner, M., Dragos, K., & Smarsly, K., 2022. Fault diagnosis in structural health monitoring systems using signal processing and machine learning techniques. In: Cury, A., Ribeiro, D., Ubertini, F., Todd, M. D. (eds.). Structural health monitoring based on data science techniques. Pp. 143-164. Cham, Switzerland: Springer, DOI: 10.1007/978-3-030-81716-9_7.
Contact
M. Tauscher, M.Sc.
Bauhaus-Universität Weimar
Chair of Computing in Civil Engineering
Coudraystraße 13 b, Room 002
99423 Weimar
Email: maria.tauscher[at]uni-weimar.de
Phone: +49 3643 58 4219